Table of Contents
1. Introduction
In the fast-paced world of modern logistics, businesses are constantly seeking innovative ways to streamline their operations, enhance efficiency, and reduce their environmental footprint. One technology that has gained considerable traction in recent years is Generative AI. This powerful tool has the potential to revolutionize the logistics industry, enabling companies to tackle the myriad of challenges they face while also promoting a greener, more sustainable future.
In this comprehensive blog post, we will explore the fascinating world of Generative AI, delving into its benefits for the logistics industry, future trends, and the risks involved. As a Green Logistics provider, Waredock is committed to staying at the forefront of these developments, ensuring that our customers and partners can make informed decisions and embrace the exciting possibilities that Generative AI has to offer.
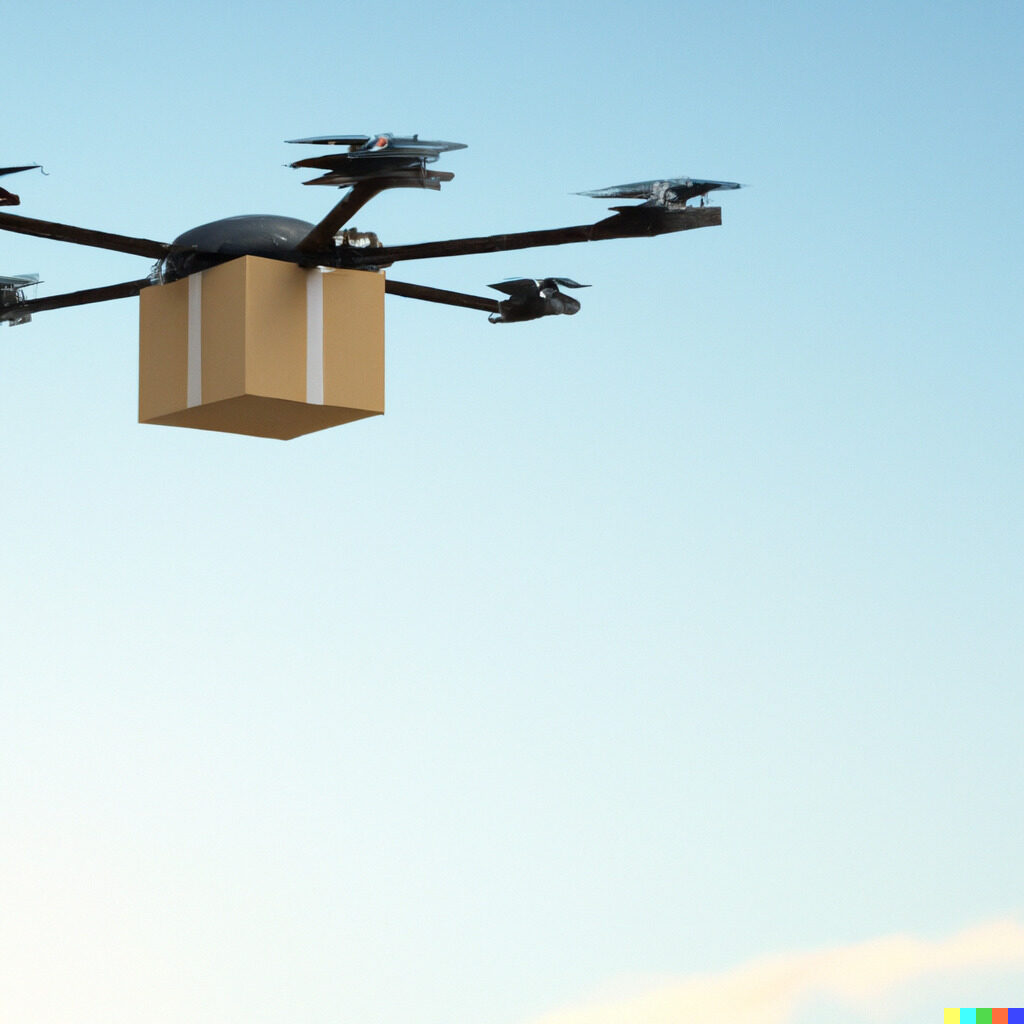
2. Understanding Generative AI
2.1. What is Generative AI?
Generative AI is a subset of artificial intelligence that focuses on creating new content, solutions, or ideas, rather than simply analyzing existing data. It employs advanced machine learning techniques such as deep learning, reinforcement learning, and natural language processing to generate outputs that were previously only possible through human creativity and expertise.
2.2. The Building Blocks of Generative AI
Generative AI models rely on two primary components: Generative Adversarial Networks (GANs) and Variational Autoencoders (VAEs). GANs consist of two neural networks, a generator and a discriminator, which work together to create realistic outputs. VAEs, on the other hand, are unsupervised learning models that use a probabilistic approach to generate new data points from a given dataset.
2.3. Key Applications of Generative AI
Generative AI has found applications in numerous industries, including art, music, medicine, and more. In the logistics sector, Generative AI can be harnessed to optimize routing, streamline warehouse operations, forecast demand, and develop eco-friendly solutions.
3. What Types of Generative AI There Are?
There are several types of generative AI, each with its own set of advantages and limitations. In this section, we will explore some of the most common types of generative AI and how they are used in logistics.
3.1 Variational Autoencoder (VAE)
A Variational Autoencoder (VAE) is a type of generative AI that is often used in image and video processing. It works by taking an input image and encoding it into a lower-dimensional representation, which is then decoded to produce an output image. VAEs are useful in logistics for tasks such as image recognition and object detection. For example, VAEs can be used to analyze images of products and classify them by their features, such as size, shape, and color.
3.2 Generative Adversarial Networks (GANs)
Generative Adversarial Networks (GANs) are a type of generative AI that is used to generate new data samples that are similar to the training data. GANs work by training two neural networks: a generator network that creates new data samples, and a discriminator network that tries to distinguish between the real data and the generated data. The generator network is trained to produce data that is similar to the training data, while the discriminator network is trained to accurately classify the data as real or fake. GANs are useful in logistics for tasks such as demand forecasting and supply chain optimization.
3.3 Recurrent Neural Networks (RNNs)
Recurrent Neural Networks (RNNs) are a type of generative AI that is used for sequential data processing, such as natural language processing and time-series analysis. RNNs work by processing sequences of data, such as words in a sentence or time-series data, and using the output of each step as input to the next step. RNNs are useful in logistics for tasks such as demand forecasting and predictive maintenance.
3.4 Long Short-Term Memory (LSTM) Networks
Long Short-Term Memory (LSTM) Networks are a type of RNN that is designed to handle long sequences of data. LSTMs are useful in logistics for tasks such as demand forecasting and predictive maintenance, where the data can be complex and difficult to analyze. LSTMs can learn to recognize patterns in data that occur over long periods of time, making them well-suited for these types of tasks.
4. The Benefits of Generative AI for Logistics
4.1 Enhanced Supply Chain Optimization
Generative AI can be utilized to optimize supply chain operations by analyzing vast amounts of data, identifying patterns, and generating optimal solutions. This includes everything from determining the most efficient transportation routes to dynamically adjusting inventory levels in response to shifting demand.
4.2 Improved Demand Forecasting
By leveraging the power of Generative AI, logistics providers can more accurately predict future demand, enabling them to make informed decisions about resource allocation, inventory management, and transportation planning. This helps minimize waste, reduce excess stock, and ensure that products are available when and where they are needed.
4.3 Streamlined Warehouse Management
Generative AI can be employed to design and manage warehouse operations more effectively, optimizing space utilization, labor allocation, and material handling processes. By automating these tasks, logistics companies can significantly reduce their operational costs and improve overall efficiency.
4.4 Reduced Environmental Impact
As a Green Logistics provider, Waredock is particularly interested in how Generative AI can help minimize the environmental impact of logistics operations. By optimizing routes, reducing waste, and promoting more efficient resource utilization, Generative AI can play a crucial role in promoting a greener, more sustainable future.
5. Future Trends in Generative AI and Logistics
As generative AI continues to advance, we can expect to witness the emergence of new trends and applications in the logistics industry. Some of these trends include:
5.1. Autonomous Vehicles and Drones
With the development of self-driving vehicles and drones, generative AI can play a crucial role in optimizing routing algorithms and navigation systems. This will enable autonomous vehicles to travel more efficiently, reducing transportation costs and carbon emissions. Furthermore, AI-powered drones can be used for last-mile delivery, especially in remote or hard-to-reach areas, improving accessibility and overall customer satisfaction.
5.2. Smart Contracts and Blockchain Technology
Generative AI can be integrated with blockchain technology to create smart contracts that automatically execute when specific conditions are met. This can lead to greater transparency, trust, and efficiency in the logistics industry by automating processes such as payment, shipment tracking, and dispute resolution. In addition, blockchain’s decentralized nature can help reduce the risk of fraud and data manipulation, ensuring a more secure and reliable supply chain.
5.3. Personalized Customer Experiences
Generative AI can help logistics providers offer personalized experiences to their customers by analyzing their preferences, behavior, and past interactions. This can result in tailored marketing campaigns, customized product recommendations, and individualized communication. By offering a more personalized experience, logistics providers can build stronger relationships with their customers and enhance brand loyalty.6
5.4. Real-time Data Analysis and Decision-Making
Generative AI can process and analyze large volumes of data in real-time, providing logistics providers with valuable insights and enabling them to make data-driven decisions quickly. This can help in areas such as demand forecasting, inventory management, and transportation optimization. As a result, businesses can respond more effectively to changes in the market and better manage disruptions in the supply chain.
5.5. AI-driven Simulation and Modeling
Generative AI can be used to create realistic simulations of various supply chain scenarios, allowing logistics providers to test and optimize their strategies in a virtual environment. This can help companies identify potential bottlenecks, inefficiencies, and risks, enabling them to develop more effective and resilient supply chain strategies.
5.6. Robotics and Automation
The integration of generative AI with robotics and automation technologies will continue to revolutionize warehouse operations. AI-powered robots can efficiently perform tasks such as picking, packing, and sorting, while machine learning algorithms can optimize their movements and coordination. This will lead to increased productivity, reduced labor costs, and improved safety in warehouses.
6. Navigating the Risks of Generative AI in Logistics
Despite the numerous benefits and exciting future trends, generative AI also presents some risks and challenges that need to be addressed by logistics providers:
6.1. Data Security and Privacy
The increasing reliance on data and AI-driven systems raises concerns about data security and privacy. Logistics providers must ensure that they have robust data protection measures in place to prevent unauthorized access, data breaches, and potential misuse of sensitive information.
6.2. Ethical Considerations
The use of AI in logistics may raise ethical questions, particularly around automation and its impact on employment. Companies need to consider the potential implications of replacing human labor with AI-powered systems and should explore ways to retrain and upskill their workforce to remain competitive in the evolving industry landscape.
6.3. Algorithmic Bias
Generative AI algorithms may inadvertently perpetuate or amplify existing biases in the data they are trained on. To minimize the risk of biased decision-making, logistics providers should actively work on identifying and addressing potential biases in their AI systems.
6.4. Legal and Regulatory Compliance
As the use of AI in logistics becomes more prevalent, new laws and regulations may be introduced to govern its application.
7. Conclusion
Generative AI has the potential to revolutionize logistics operations. By analyzing large datasets and identifying patterns, businesses can make informed decisions that lead to better outcomes. From demand forecasting to supply chain optimization, generative AI can be used in various ways to improve logistics operations. There are several types of generative AI, each with its own set of advantages and limitations. By understanding the different types of generative AI and their applications, businesses can choose the right technology for their specific needs and achieve greater success in their industry.
NOTE: The whole article was written as an experiment by ChatGPT4 and does not necessarily reflect the opinions of Waredock Logistics. Learn more about Waredock Green Fulfillment Network here.